Predicting Fatigue in an Unpredictable Future
- Jaime K. Devine, PhD
- Apr 15, 2021
- 6 min read
Updated: Aug 24, 2021
Things that we had considered predictable and unwavering for generations, like commuting to work, or being able to go to a grocery store, changed in 2020. People around the world are beginning to emerge from the pandemic like the brood X cicadas currently digging their way out of my lawn after 17 years of underground living, but we may never lose this new fear of uncertainty that COVID-19 instilled in us. Is another lockdown around the corner? How will the ‘new normal’ change international business operations? How can we predict risk in an unpredictive future? Perhaps this sense of Schrodinger’s dread is acutely annoying to those of us in the realm of predictive modeling.
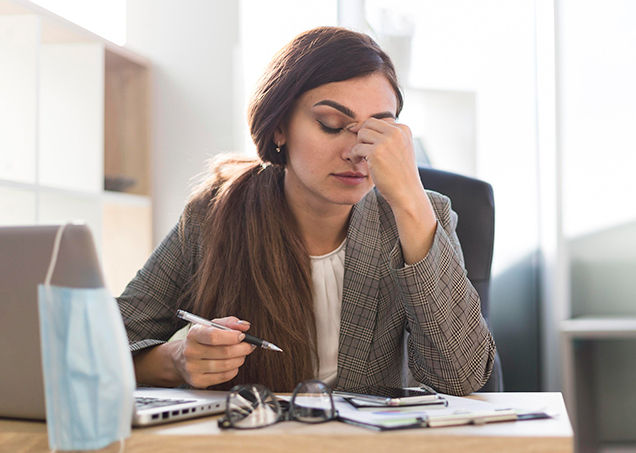
Prediction models rely on previously-collected data. Last spring, if you were concerned how extended sit times as airplanes waited to clear travel restrictions may be affecting a pilot’s fatigue, or how working overtime in a newly-minted COVID ward might be related to alertness in nurses, resources for answering those questions were severely limited. Pandemics have happened before 2019, but preparing for the outbreak of disease was previously a job for epidemiologists or healthcare providers. If anyone was collecting data on fatigue or sleep behavior during the 1918 influenza pandemic, their findings have been long forgotten by the 21st century.
One year deep into a post-COVID world, however, and the search results are growing. Operational fatigue researchers have done their best to document what happened during the COVID-19 pandemic, and their findings can help inform fatigue modeling moving forward. A biomathematical model cannot tell you whether an industry will be forced to adapt to new paradigms of work schedule management, but understanding the capacity of humans to obtain rest under extreme and unexpected schedule changes can help us determine which new paradigms may be associated with fatigue risk.
An interesting side effect of the COVID-19 lockdowns was a global change in sleep behavior. Yuksel et al. reports in their 2020 article in the Journal of Sleep Health, entitled “Sleeping when the world locks down: Correlates of sleep health during the COVID-19 pandemic across 59 countries”, that a third of respondents reported having more sleep disturbances. Job-related stress factors like being laid-off or transitioning to work-from-home were strongly correlated with increases in sleep problems in this sample as well. Fatigue or diminished sleep quality related to to the stress of the pandemic has been consistently shown in general and operational populations (1-4).
Of particular interest, a study by Wilson et al., entitled “The effectiveness of a 17-week lifestyle intervention on health behaviors among airline pilots during COVID-19,” examined how educating pilots about sleep hygiene and setting personalized goals could influence sleep quality during COVID-19 lockdowns. They found that the intervention resulted in better sleep quality at the end of lockdown compared to pilots who received no sleep hygiene training (3). Pilots who did not receive the sleep hygiene intervention reported worse sleep quality at the end of the lockdown period compared to their own report of sleep quality at the beginning of lockdown. That is to say, lockdowns resulted in a worsening of sleep quality in pilots which could have been prevented by a lifestyle intervention. These findings suggest that fatigue risk can be minimized through proper training and organizational support.
Calls for better organizational management of fatigue have been particularly loud in the domain of healthcare workers over the past year (5,6). Unlike transportation industries, the healthcare field hasn’t yet adapted biomathematical modeling for fatigue risk management. Some providers have begun to take initiative to include biomathematical modeling for fatigue risk management (7-10), but healthcare providers are facing an onslaught of logistical nightmares at the moment. Now may not be an easy time for individual organizations to exercise “initiative”, and there are no central industry standards for fatigue beyond limitations to working hours. Healthcare organizations in a post-COVID era could benefit from a systematic adoption of fatigue modeling in order to mitigate risk in their workers and develop innovative solutions beyond limiting hours of work.
You may be asking if the models are still relevant, given that so much has changed. Schedules may have changed, stress levels have changed, but the circadian rhythm and the human biological need for sleep persists. Not all biomathematical models rely on sleep as an input or utilize a sleep prediction algorithm like SAFTE-FAST’s Auto-sleep (11-13), but it is reasonable to expect that the ability of workers to obtain sufficient rest within the parameters of a work schedule will be the same whether that work schedule was from 2019 or 2022. Auto-sleep was recently used to forecast fatigue risk prior to conducting direct COVID-19 humanitarian flights between Brazil and China. The results of the sleep predictor were then compared against objective actigraphy and sleep diary provided by the pilots during these unprecedented ultra-long-range missions. In a head-to-head comparison, Auto-sleep predictions of sleep duration and effectiveness were as good as either diary or actigraphy records of sleep (14). This indicates that the ability of Autosleep to predict sleep is resilient in the face of unprecedented operations.
Biomathematical modelling remains a tool which uses knowledge about human biology to present objective data about potential areas of risk. When it comes to actually managing that risk in a post COVID-19 world, much of the eventualities will lie in how industries adapt their scheduling practices. Conducting more ultra-long-range hub-and-spoke flight might be good from a business perspective, for example (15), but doesn’t account for the human factor. Accounting for human factor limitations early on, such as by prospectively modeling fatigue risk for proposed schedules beforehand, can help reduce the ominous shadow of uncertainty which is currently hanging over any expectations of a return to normalcy after the pandemic.
Footnotes
Comments